A fundamental change in media consumption behaviour coupled with quantum leaps in AI technology has made AI-translated captions a popular and powerful choice for live events of all shapes and sizes. Interprefy introduced them 2022, and they are also available on Microsoft Teams, and even Zoom: auto-generated, multi-lingual subtitles for live meetings. This technology makes it possible for users to understand, even if they don't know the language the speech is on.
But how accurate are they? There is no simple answer. The results highly depend on the chosen approach and engines used, the specific language combination, as well as properties of the audio (speaker accent, audio quality, etc.). And the simple truth is that there is no definite method of measuring translation accuracy.
People in the translation industry describe quality in various ways. When attempting to come up with an objective measure, a group of researchers admitted that they couldn't even agree amongst themselves on how "translation quality" should be defined.
Let's take a closer look at why translation quality is so hard to measure and how we can get closer to measuring machine-translated captioning quality.
How automatic subtitles in multiple languages work
"Auto-translated", "machine translated", and "AI-translated" captions or "multilingual subtitles" are closed captions that provide users with real-time subtitles alongside speech in a different language. They are created from the source audio by using either a combination of automatic speech recognition and machine translation technologies that produce a translated text of the transcript, or an AI-based solution that directly converts the audio in the source language into text (or even spoken speech) in the target language.
Measuring translation quality
Language is highly complex and so the quality of a translation is often subject to interpretation. One might assume that quality issues occur when a translator or machine makes a mistake. However, it is much more common that what is seen as translation quality issues, is a subjective assessment.
The Multidimensional Quality Metrics (MQM) framework, a project headed by the European Commission, provides a "functionalist" approach that categorises quality issues:
- Accuracy
- Style
- Fluency
- Locale Conventions
- Terminology, etc.
That's why organisations often provide translators with style guides, glossaries and ideally even build up a translation memory, to get consistency across their translation works that matches their needs.
Measuring the quality of translation is a matter of evaluating how useful the translation is, and how well it fits its purpose.
Machine translation quality for live captions
Machine translation has been around for over 60 years, and today machines and humans coexist. But over the recent two decades, Language Service Providers (LSPs), translation agencies and freelancers have adopted machine translation to improve productivity and reduce cost, thanks to the rapid evolution of machine translation quality.
Not all machine translation engines are equal
Today there is a multitude of text-to-text translation engines available, like Google Translate, DeepL Translate, or Microsoft Translator, as well as several types of machine translation: rule-based, statistical, adaptive, and neural. Most services have begun moving towards the last one, as neural machine translation has proven to be powerful in producing exceptionally satisfactory results and quickly bridging the gap between humans and machines for certain types of texts.
Different translation engines and different types of machine translation produce different results. One engine might even do an exceptional job for one language combination but produce useless results for others.
Real-time vs post-editing requirement
Because most written translation doesn't have to be finalised instantly, the machine translation output for websites or documents is reviewed and post-edited by professional translators before publishing. Therefore having the best engine is a real timesaver, but not essential.
Live multilingual subtitles, however, need to be delivered in real-time, without the possibility of human intervention before the user reads them.
Therefore it's crucial that the best-performing engines and engine combinations are used and that audio input quality is optimal. If for instance, a speaker has a heavy accent and uses a bad microphone, even the best solutions might produce less-than-great multilingual subtitles.
The Interprefy approach: Benchmarking solutions and optimising input audio
Instead of using one single machine translation engine, Interprefy's AI delivery team continuously benchmarks leading translation solutions as well as combinations of voice recognition and machine translation solutions for specific language combinations.
We collaborate with world-leading research institutions to develop and continuously improve a proprietary and automatic benchmarking process for live multilingual subtitles. Alexander Davydov, Head of AI Delivery at Interprefy
"We use large sets of diverse audio data and we take the output from various translation systems and combinations of systems and compare it with translations produced by professional translators, validate them and rank them by accuracy", Alexander explains.
The chart below illustrates the benchmarking results for four languages translated from the same source language. As you can see, no single solution provides consistent quality for all four language pairs.
But even if you have the most sophisticated solution, quality can still suffer, if the input quality is low.
Sound quality is a key factor that affects not only the quality of the AI output but also the interpreters’ health and ability to perform, as well as the audience’s understanding and engagement. That is why at Interprefy we continuously strive to improve audio quality by providing event organizers and speakers with useful guidelines, facilitating tools for speakers to test their sound quality, and even developing an audio enhancement tool, Interprefy Clarifier.
Additionally, our expert staff work with our clients to optimise the system to get brand names, acronyms, and more right.
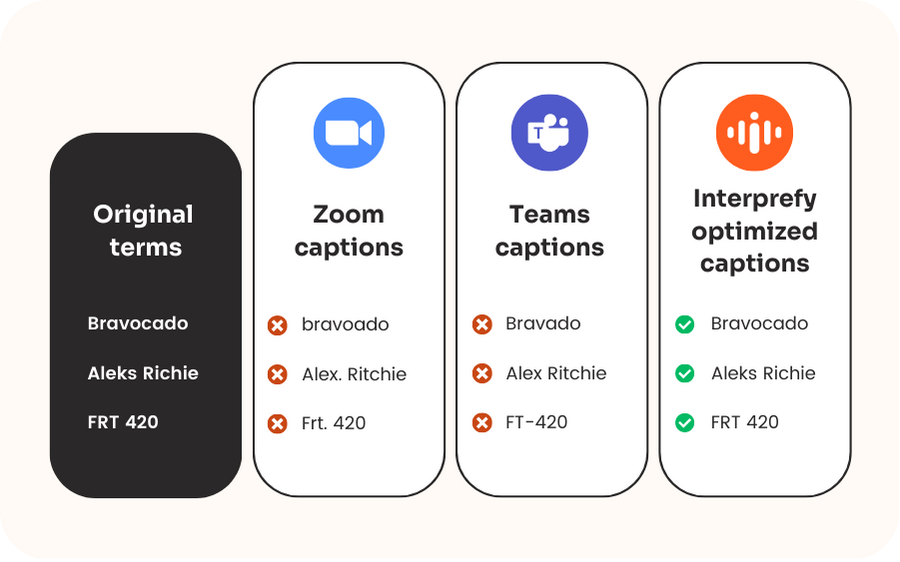
Want to carry out your own quality assessment?